In our survey of vocational school teachers in summer 2020, it emerged that the teachers surveyed would like to see case studies from business practice on how to deal with AI. At the end of 2020, the project partners from ITB therefore met with the team from the company TRILITEC. In this article, you will discover what the company does and how AI is used in the process.
What is TRILITEC?
The start-up launched in 2015 with the topic of applying millimetre wave sensor technology in various areas. In 2017, the idea of testing this millimetre wave technology on harvesters and combine harvesters for the detection of foreign objects was inspired by tips from farmer friends. This is intended to detect foreign bodies that are not perceptible to the machine operator, such as stones, rubbish, animals and/or metal parts that may be in the field, promptly. Appropriate visual and acoustic warning signals on the operator’s control panel should make it possible to stop the machine in time. This can prevent the harvester from suffering extensive damage from foreign objects. According to the company’s founders, the insurance industry’s damage reports show that in many cases, damage amounts as high as six digits can accumulate. Damage to harvesters by foreign objects is therefore a major real problem facing many different institutions in the agricultural sector, such as combine harvester manufacturers, the insurance industry, contract threshers, farmers and, last but not least, the forestry industry. The system is currently in the test phase. Discussions are in progress with machine manufacturers on how the implementation and corresponding trials can look like. In addition to the agricultural sector, the millimetre wave technology is also being tested by the company in the food sector, e.g. for quality assessment (structure of meat, the shape of rolls, etc.). and detection of foreign bodies in food products. This technology is not new; it is used, for example, in the nude scanners in the security area of airports to identify risky objects hidden on passengers’ bodies more quickly.
What role does Artificial Intelligence play in this?
For the system, which is based on millimetre-wave sensor technology, to be able to distinguish a “harmless” corncob in the field from a foreign object that is causing trouble, a lot of data must first be collected. In this data, characteristics of objects, e.g. the corncob or the metal part, must then be identified so that the system can strike in case of danger. There are two ways to collect the data:
Scenario 1: Drive over the same field several times with the millimetre wave sensor system. This can produce quite a lot of data, but it only relates to the tested field and does not take into account different cultivation options of e.g. corn. So the collected data represent the tested field very validly but are not optimally transferable to other fields, which means that the warning cannot be targeted precisely.
Scenario 2: Many different arable fields are selectively driven through in order to better take into account the variance of the individual fields, e.g. corn cultivation techniques, in the data and to have the system sound an alarm in the event of “real” foreign objects in the driver’s cab. This data generation scenario is significantly more complex, but also more precise.
From this collected data, the system should then learn when to sound the alarm and when not to. The basic ‘problem’ with data generation is that very little data is collected from foreign objects, but mainly “good data”, i.e. fields on which there are no foreign objects.
What role do ethical considerations play in data collection? The company founders report that the issue of ethics is present even though no personal data is collected. Nevertheless, says one of the brothers, you always have to ask yourself what data is being collected, e.g. tracking the working hours of the harvester drivers. That sounds unproblematic at first. However, if this data were to reach the employer, it would be personal information, albeit indirectly. According to the brothers, such scenarios must and will be considered.
How do employees learn at TRILITEC? Which competencies are important for the work (now and in the future)?
The brothers taught themselves about AI and machine learning rather autodidactically. Learning in everyday work mainly takes place through exchange and collaborative work and development between the five developers employed in the company and with the inclusion of students. Staff expertise with databases is important, as it often involves competent handling of large amounts of data. How do I handle data? How do I store data systematically? How do I analyse the data?
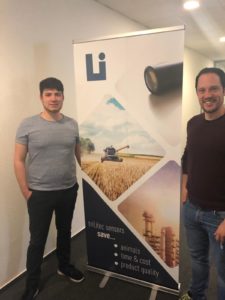
The company founders report that it has been quite difficult so far to recruit specialists who have know-how in the field of AI or machine learning as well as millimetre wave technology. This is another reason why they work a lot with the university and the college – to build up the next generation. In particular, there is close cooperation with students of the corresponding courses of study in electrical engineering and computer science. The company does not yet work with vocational training institutions. However, they emphasise that when the product goes out of development, IT specialists (from vocational training schools), in particular, will be very interesting for the company because these people already have a lot of practical experience with automation systems. In this respect, the company can very well imagine looking for apprentices in these special fields and providing them with qualified training in the work and order process and using them as quality managers at the interface between production and development.