AI and Assessment
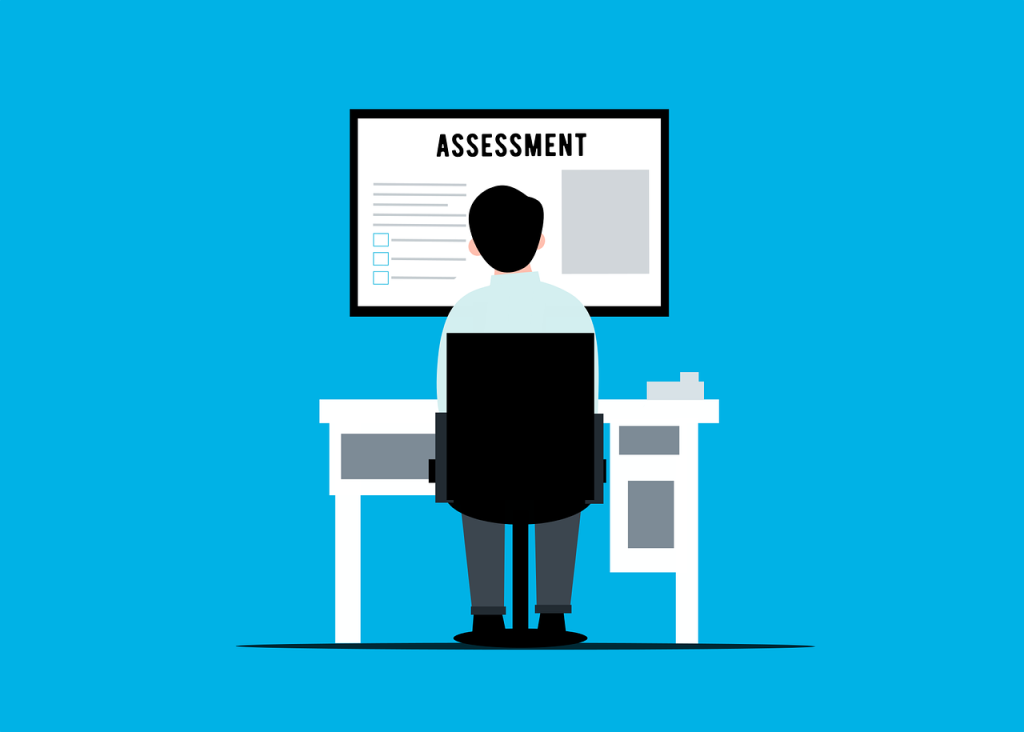
Maybe the panic over the impact of AI on assessment in education has died down a little, but it has been useful in that it has focused attention on the puropse of assessment and the pedagogic approaches to assessment. Simon Brookes, Executive Dean, Faculty of Creative & Cultural Industries, at the University of Portsmouth in the UK has started a new blog series on Rethinking Assessment in the Age of AI. His latest post features insights from the University of Melbourne's Centre for the Study of Higher Education. Their recent guide, "Rethinking Assessment in Response to AI" (pdf) offers a thoughtful approach to redesigning assessments that maintain academic integrity without sacrificing pedagogical value, he says.
The guide includes seven critical strategies for improving assessment design and integrity:
1. Shift from product to process: Focus on evaluating students' thinking processes and problem-solving approaches rather than just the final output. This could involve asking students to maintain learning journals, document their research process, or explain their reasoning in solving problems.
2. Incorporate evaluative judgement tasks: Ask students to review or evaluate work against set criteria, encouraging higher-order thinking skills. This might include peer review exercises, critiquing published works, or assessing case studies against industry standards.
3. Design nested or staged assessments: Create assignments that build on each other throughout the semester, allowing for feedback and adaptation. For example, a research project could be broken down into proposal, literature review, draft, and final submission stages, each informing the next.
4. Diversify assessment formats: Use various modalities, such as videos, blogs, podcasts, and animations, which are less susceptible to AI generation. This not only makes cheating more difficult but also allows students to develop a broader range of communication skills.
5. Create authentic, context-specific assignments: Design tasks that mirror real-world scenarios or are highly specific to the subject matter. This could involve analysing local case studies, solving problems specific to your discipline, or applying theories to current events.
6. Include more in-class and group assignments: Incorporate collaborative learning and reduce opportunities for individual cheating. This might involve group presentations, debates, or problem-solving sessions during class.
7. Use oral interviews: Test understanding through verbal responses to unpredictable prompts, making it difficult to use AI. This could range from viva voce examinations to informal discussions about a student's work process.