AI and Education: Agency, Motivation, Literacy and Democracy
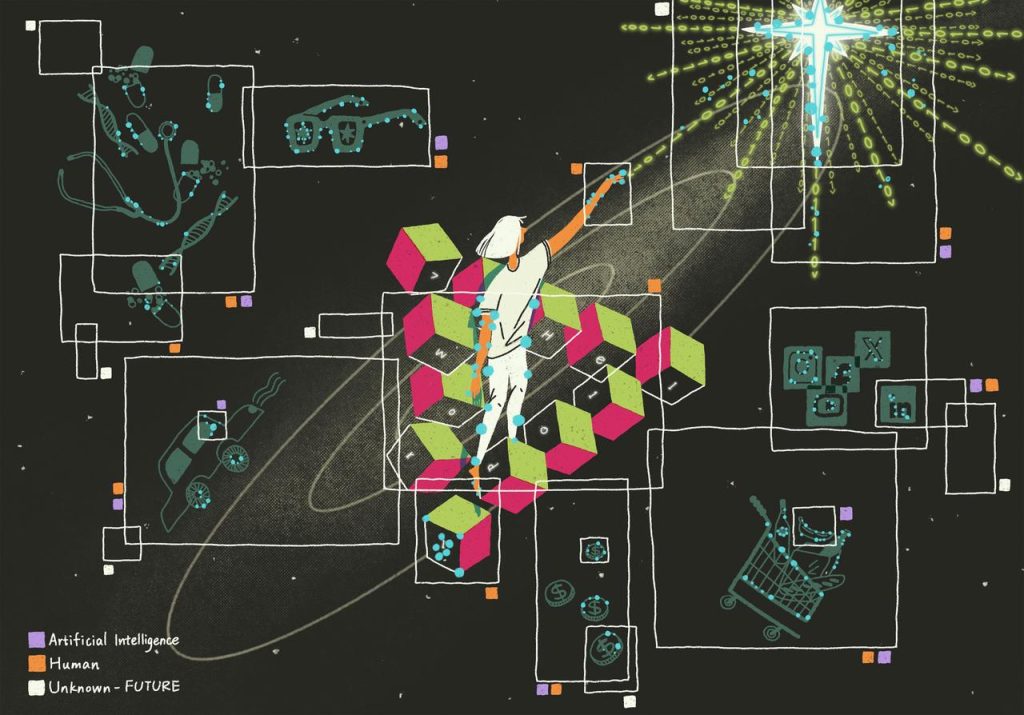
Graham Attwell, George Bekiaridis and Angela Karadog have written a new paper, AI and Education: Agency, Motivation, Literacy and Democracy. The paper has been published as a preprint for download on the Research Gate web site.
This is the abstract.
This paper, developed as part of the research being undertaken by the EU Erasmus+ AI Pioneers project, examines the use of generative AI in educational contexts through the lens of Activity Theory. It analyses how the integration of large language models and other AI-powered tools impacts learner agency, motivation, and AI literacy. The authors conducted a multi-pronged research approach including literature review, stakeholder interviews, social media monitoring, and participation in European initiatives on AI in education. The paper highlights key themes around agency, where AI can both support and challenge learner autonomy depending on how the technology is positioned and implemented. It explores the complex relationships between AI, personalization, co-creation, and scaffolding in fostering student agency. The analysis also examines the effects of generative AI on both intrinsic and extrinsic motivation for learning, noting both opportunities and potential pitfalls that require careful consideration by educators. Finally, the paper argues that developing critical AI literacy is essential, encompassing the ability to understand AI capabilities, recognize biases, and evaluate the ethical implications of AI-generated content. It suggests that a broader, more democratic approach to curriculum and learning in vocational education and training is necessary to empower students as active, informed citizens in an AI-driven future. The findings provide an approach to the complex interplay between generative AI, learner agency, motivation, and digital literacy in educational settings, particularly in the context of vocational education and adult learning.